Day :
- Data Mining Applications in Science, Engineering, Healthcare and Medicine Big Data in Nursing Research | Big Data Technologies | Big Data Analytics | Big Data Algorithm | Big Data Optimization | Forecasting from Big Data | Big Data Applications, Challenges and Opportunities | Data Mining and Machine Learning | Data Mining Methods and Algorithms | Data Mining Tools and Software | Data Mining Tasks, Processes and Analysis | Data Privacy and Ethics | Data Warehousing | Artificial Intelligence | Cloud Computing | Social Network Analysis | Business Analytics | Internet of Things (IOT)| Open Data | New Visualization Techniques | Search and Data Mining | Frequent Pattern Mining | Clustering | Complexity and Algorithms | Nanoinformatics | Renewable Energy |Forecasting with Big Data
Session Introduction
Paria Soleimani
South Tehran Branch, Islamic Azad University,Tehran, Iran
Title: Identifying the effective factors on brand value (Case study: Automobile companies of Tehran Stock Exchange)
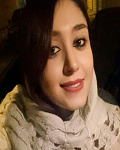
Biography:
Abstract:
Karmen Kern Pipan
Ministry of Public Administration, Republic Slovenia
Title: Supporting improvements in decision making process using big data analytics in Ministry of Public Administration of Republic Slovenia
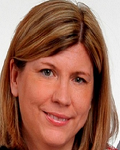
Biography:
Abstract:
Gyeongseok Oh
Sam Houston State University, USA
Title: Crime Prediction Using Administrative Big Data and Machine Learning
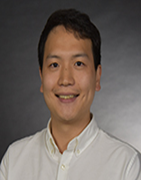
Biography:
Gyeongseok (Jason) Oh began the SHSU Criminal Justice Ph.D. program in the Fall of 2017. In 2016, he graduated from Florida State University with an M.S. in Criminology and Criminal Justice. He also received an M.A. in Criminal Justice from Yongin University. Before pursuing graduate studies, he worked as a detective in the Korean Police Agency for six years after obtaining his Bachelor’s degree from Korean National Police University. He is currently working on the research project entitled, “Social media analysis of neighborhood sentiment and its impact on crime patterns” with Dr. Yan Zhang. His primary research interests include crime analysis using Big Data and machine learning, policing, and biosocial criminology.
Abstract:
It is indisputable that machine learning techniques and big data analysis have become the main topics in almost all discipline of science and industry during the past decade. Concurrently, numerous governments in the world are collecting enough amount of administrative data that can be analyzed by machine learning techniques to investigate the causes of social phenomena and to improve the efficiency of public administration. Despite the data analytic techniques and the capability of data storage have been remarkably improved, a large number of scholars in the field of social science hold conservative perspective on applying machine learning and big data analysis to explaining social phenomena. The goal of this study is to fill the void by providing empirical evidence. The present study will attempt to examine the validity of using administrative big data to predict crime incidents. Records of calls for service through 311 mayor’s hotline system in Houston, Texas and the official crime reports of Houston Police Department were examined to assess whether signs of physical decay and the presence of social nuisance predict the crime incidents at neighborhood level. The results of this study will corroborate the Broken Windows Theory and present new windows to explore the causes of crime. Several policy implications for government and police administrators will be developed and discussed.
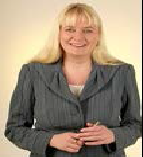
Biography:
Prof. Petra Perner (IAPR Fellow) is the director of the Institute of Computer Vision and Applied Computer Sciences IBaI. She received her Diploma degree in electrical engineering and her PhD degree in computer science for the work on “Data Reduction Methods for Industrial Robots with Direct Teach-in-Programing”. Her habilitation thesis was about “A Methodology for the Development of Knowledge-Based Image-Interpretation Systems". She has been the principal investigator of various national and international research projects. She received several research awards for her research work and has been awarded with 3 business awards for her work on bringing intelligent image interpretation methods and data mining methods into business. Her research interest is image analysis and interpretation, machine learning, data mining, big data, machine learning, image mining and case-based reasoning.
Abstract:
Ho-Chan Kwak
Korea Railroad Research Institute, Korea
Title: Prediction of Hourly Floating Population based on Mobile Phone Data in Korea
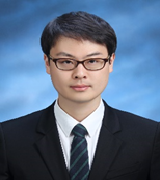
Biography:
Abstract:
Jiyoung Song
Korea Railroad Research Institute, Republic of Korea
Title: Development of estimation model of the infectious diseases spread using big data
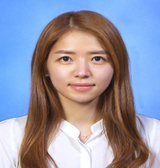
Biography:
Abstract:
A system to quickly estimate the spread of infectious diseases is needed due to the fear and threat of infectious disease spread all around the world. It is necessary to simulate the disease spread estimation model that is specific to each region and environment of each country. Korea needs environmentally optimized disease spread simulation technology. The combination of real-world data on population and human mobility and disease spread models is a global trend to enhance predictive power. Utilization of Big Data makes it possible to improve the reality of infectious disease spread model. Government of Korea Activating the opening and sharing of public information and we have environment in which a variety of evidence can be obtained to increase the reliability of disease spread estimation.
Irfan Mohiuddin
King Saud University Riyadh, Saudi Arabia
Title: Quality Assessment of User Generated Content on Twitter–A Deep Learning Based Approach
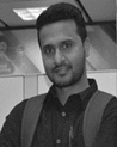